The AI bubble is going to burst-- corporate greed is the cause
The public is getting tired of hearing about 'AI'. Big tech is to blame. What happens next?
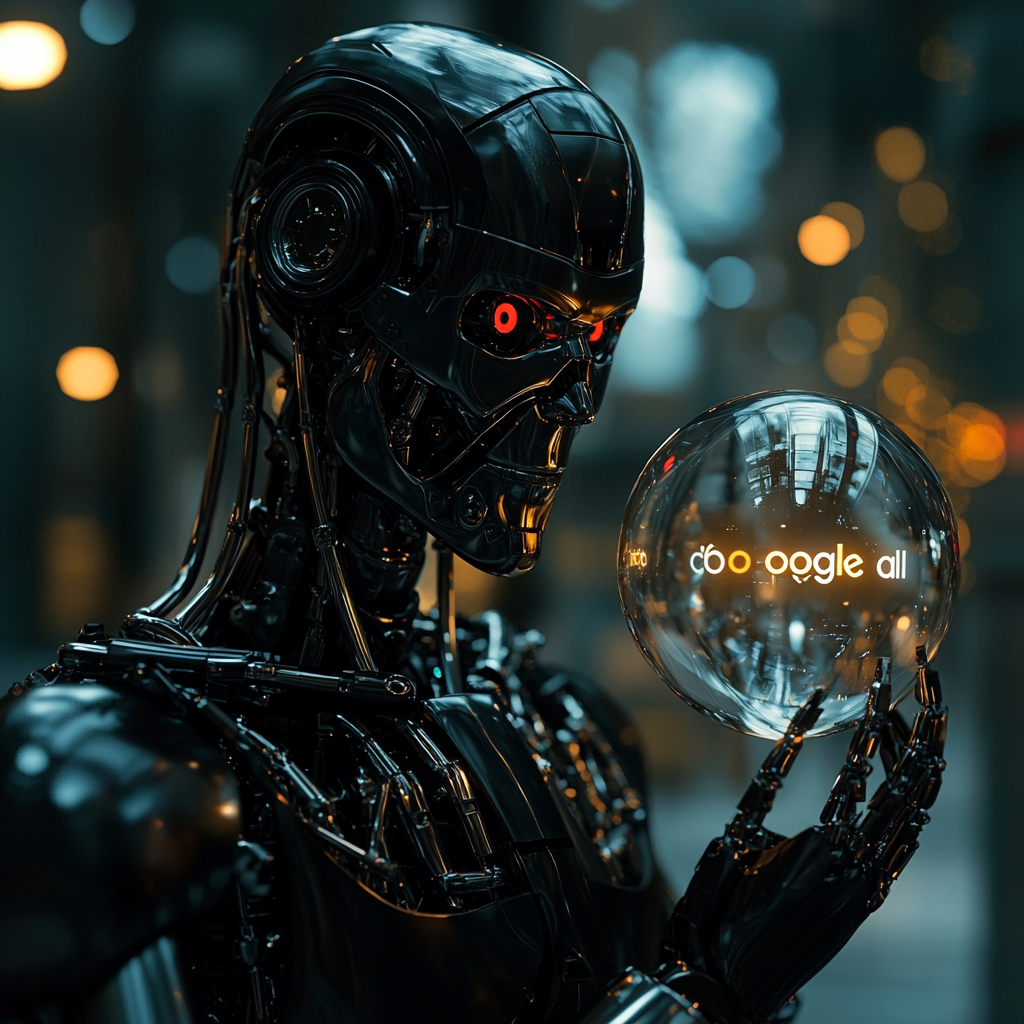
In February 2023, ChatGPT exploded into the mainstream, after a slow start. ChatGPT was an evolutionary leap in how humans interacted with computers. Instead of having to write machine specific instructions (e.g., code), a person now had the capability of querying a machine with simple language. This problem, previously rooted in the NLP space (natural language processing, a discipline within the broader machine learning space), was seemingly solved over night by a 'new' type of machine learning application, large language models.
Interestingly, these large language models also seemed to demonstrate capabilities in reasoning across vast swathes of digital information, arriving at fascinating conclusions, derived from massive, mindbogglingly large datasets. However, at its core, these LLMs were just demonstrating something expected: the ability to thread between multiple contexts, subjects, and disciplines, to arrange letters and characters into a sequence of strings that were most probable given what they were being asked.
Anybody who had the opportunity to play with the earlier GPT models from OpenAI, like GPT-2 and GPT-3, immediately recognized what was happening under the hood. However, to the rest of the world, the product interface of 'ChatGPT' was a magic black box that let you communicate with an 'artificial intelligence', an entity that somehow was able to take any question or thought and come back with an intelligible answer. It's easy to understand why, when that happened, people misunderstood what these models were, and The Great Conflation happened. ChatGPT went viral. The technology story, previously understood by those who had been previously using it, became obfuscated behind walls of propaganda and marketing from companies like OpenAI, Google, Microsoft, and others.
The propaganda benefitted the narrative that OpenAI, Google, and now other well-known entrants like Anthropic, had been working on building a true 'artificial intelligence', a SkyNet-like being that we must all be afraid of. However, while it is true that AGI (Artificial general intelligence, what you'd typically see portrayed in something like a science fiction novel or movie) was being researched and worked on, the GPT models and the ChatGPT product interface certainly were not true representations.
In the simplest and most reductive terms, this new wave of 'AI' is simply providing the most statistically relevant next character or letter, based on what you've provided. It's not reasoning or thinking about what you've asked it – large language models have no capacity for thinking or reasoning – but rather, estimating based on its corpus of knowledge (which is insanely massive, 'The Internet and Beyond' in scope), what the next most likely letter or character will be.
Disclaimer: This is not to say that these large language models aren't amazing, and useful, and powerful, and shouldn't be explored, but rather to level set on what's actually happening here. Once you understand that it is the letter/word (more specifically it's an amalgamation of these things, generalized as a 'token') that you wrote being predicted, you can start seeing beyond the veil. If you provide legal-ese to the model, it will find a more cogent, legal-ese type response. If you provide a simple statement, it will find a simple statement. Large language models, by design, provide output relative to your input.
If I say, "I am rock. Rock be good. Love rock.", this is interpreted entirely different than if I say "I have certain amounts of iron in my blood. Iron is an important nutrient for my body. I should get more iron, because it is good for me."
There has been an incredible amount of product work done by OpenAI recently to obfuscate this fact, by modifying the interface (ChatGPT) and underlying data within these models to make it seem as if there is an underlying intelligence, but this is smoke and mirrors. This is good product work and design, backed by a sophisticated model, that presents a powerful illusion. But that's what it is: a good product backed by an interesting technology. It is not an artificial intelligence; it is not a general-purpose problem solver.
Large language models are one of the next big things– no doubt. The recent surge in popularity, however, is not driven by the factual utility of this models, but the expected output they will provide in the future. Large language models are being marketed as 100x productivity increasers, as tools to reduce both time spent, and resources needed to accomplish audacious goals.
Example: There are a multitude of projects that advertise providing the "world's first AI software engineer", as an out-of-the-box solution for all your software development needs. ChatGPT, even by itself, is pretty capable of producing code. And it does a great job of demo'ing these capabilities too, letting you produce a workable app on the web in just a few quick prompts and questions. You can see from the demo that, undoubtedly, this is faster than hiring somebody or writing the code yourself for this application. However, these large language models suffer from a very human issue: the 80/20 rule, or the Pareto principle, which states "that for many outcomes, roughly 80% of consequences come from 20% of causes".
Software developers already know this, all too well. It can be deceptively simple to spin up a greenfield application, and often tempting to rewrite an entire codebase, if only to escape the painful last 20% of a project that is either the bulk of the most complicated/frustrating work to do, or the essential work that can cripple the project if not done well. ChatGPT may help you with the first 80% of the work, but inevitably you find yourself mired in the last 20%, wherein you spend the same (or more time) working to get over the finish line, even with the assistance of ChatGPT. ChatGPT, then, is simply an alternative way to get something done, and not an evolution.
And this is why the bubble is going to burst.
Large language models are not productivity increasers, which is what big organizations and corporations would like, as this allows them to 'do more with less' but rather knowledge democratizers, wherein more people now have access to a set of tools and knowledge that they never had before.
Example: A scientist working at a concrete company wants to develop an internal application to help out their broader team. The scientist is proficient in R and Matlab but has no familiarity with the broader web ecosystem. They don't know how to host a cloud application, create a nice frontend for a website, and all the other tasks associated with that. However, using ChatGPT, they are able to accomplish these goals. This same task may have been delivered faster by a dedicated software developer (or approximately the same amount of time), but these types of resources are often not available to somebody working within an internal team. This is an empowerment tool, not a productivity tool.
The marketing and sales organizations that are selling the dream of AI as ways to reduce the number of labor/resources needed are fundamentally backwards. Large language models are a way to augment new skills on to an existing workforce that was capable of learning those skills but fundamentally did not have them to begin with. This means that more people will be capable of greater things, be able to realize more ideas, but not necessarily work faster and/or harder on existing things.
Final thoughts
When you have a tool that makes it easier for everybody to do new things, this is often not aligned to corporate interests. What a corporation often wants is purpose-built people, in specific functions, operating most productively. One cog touches another cog and then another, and the machine is spinning faster, and producing more. Corporate assembly at its finest.
Large language models have the opportunity to be democratizing. It can enable a new generation of entrepreneurs, creators, learners, and others, to do new and big things previously unavailable to them. In my next post, I'll talk about how I think we can use these tools to achieve escape velocity in our lives, for whatever it is what we want to do.